Snow hydrologic modelling for operational reservoir management in high-alpine catchments
SUPERVISOR: Karsten SCHULZ
PROJECT ASSIGNED TO: Thomas PULKA
Seasonal snow is an essential component of the hydrologic cycle and dominates runoff regimes of high-alpine catchments. Information on snow cover dynamics and snow melt in such catchments is a prerequisite for the operation of storage hydropower plants, the mitigation of floods from snowmelt peaks and heavy rainfall events or the characterization of hydro-ecological regimes in Alpine and lowland river system.
Spatially distributed hydrological models are important tools to simulate the complex hydro-meteorological process in high-alpine catchments. Snow hydrological process description are often based on simple temperature index-based routines that might show deficits, as they are often unable to sufficiently represent the complex physical processes of seasonal snowpack dynamics, e.g. under Föhn conditions, topography driven melt out patterns in spring or the wind redistribution of snow. The application of complex physically-based models can solve these limitations to a certain extent, but demand a large number of input parameters and will be limited by computational demands and long run times, which might hinder the use in real-time systems.
Thus, an optimal balance between increasing model complexity and computational demand for operational applicability is one of the current challenges not only in Alpine hydrology, but also in other environmental disciplines. Therefore, I will investigate the performance of different complex models describing snowpack dynamics for high-alpine headwater catchments in order to identify the most effective compromise between performance, complexity and costs.
An initial test-site will be the headwater catchment of the Kölnbrein reservoir in the Malta Valley, which is one of the largest reservoirs in Austria, with a capacity of 200 million m³. The highly complex physically-based model SNOWPACK/Alpine3D (Bartelt and Lehning, 2002; Lehning et al., 2006), the spatially explicit and hydrology-oriented cryospheric model Snow Multidata Mapping and Modeling (S3M) v5.1 (Avanzi et al., 2021) and the conceptual precipitation-runoff model COSERO (Nachtnebel et al., 1993; Herrnegger et al., 2018; Wesemann et al., 2018) will be tested and performance criteria will be compared. To investigate different scales of model performance, the spatial resolutions will be varied between 50, 250 and 1000 m. In addition, different sets of meteorological drivers will be analysed, including the INCA (Integrated Nowcasting through Comprehensive Analysis; Haiden et al., 2011) precipitation analysis product provided by ZAMG (the Austrian Central Institute for Meteorology and Geodynamics), ZAMG station data recordings from several stations in the larger area (approx.. up to 25 km distance), and the latter in combination with in situ measured data at meteorological stations operated by VERBUND AG within the catchment or close by.
At specific dates, the simulated snow cover extent and the snow height will be evaluated with spatially distributed snow height observations stemming from UAV-surveys and satellite-based data. In addition, in-situ snow height and discharge measurements are available for model evaluation. As a further possible evaluation information for the snow-hydrologic modelling, the utilization of optical imagery data from webcams and terrestrial LIDAR in the test site will be evaluated. The detailed analysis of the simulated snowpack in combination with the validation data will be used to compare the three models and to show the differences of their simulations.
Due to the heterogeneity of physiographic properties of high-alpine catchments mainly due to its complex topography, the accumulation, settlement and ablation processes of snow are spatially unevenly distributed. The identification of the physiographic catchment characteristics which have a pronounced influence on the aforementioned snow processes supports the understanding of the hydrological behaviour of the catchment and also the advancement of the hydrological model in use. The complex physically-based model Alpine3D is able to simulate, e.g. wind drifted snow and the topography based heterogeneity of snow melt out in a high resolution, however demanding high computational effort. Machine learning (ML) algorithms are able to learn complex non-linear behaviour and have shown to perform very well in hydrological studies. Therefore, ML will be used for the regionalisation of catchment characteristics (e.g. clustering) and to predict catchment runoff (e.g. Long Short Term Memory Neural Network; see Kratzert et al., 2018; Kratzert et al., 2019 or Feigl et al., 2021). Since the trained LSTM demands less computational effort, this approach can be especially valuable in the snowmelt period and for heavy rain on snow events and therefore support reservoir management and flood mitigation.
The analysis of different meteorological driving data, different regionalisation strategies and the application of different model types will increase our knowledge of the snow-hydrologic processes in the high-alpine headwater catchments of the Kölnbrein reservoir. The insights of the analysis of the different snow-hydrologic models will be used to advance the snow routine of the conceptual hydrologic model COSERO and thereby improve reservoir management and flood mitigation.
Key points
- Investigation of different snow-hydrologic model complexities with regards to model performance and computational demand
- Evaluation of different spatial resolutions and different meteorological driving data
- Exploration of a possible application of remote sensing techniques in high-alpine catchments
- Application of ML methods for regionalisation and prediction problems
- Advancement of the snow routine of the conceptual hydrologic model COSERO
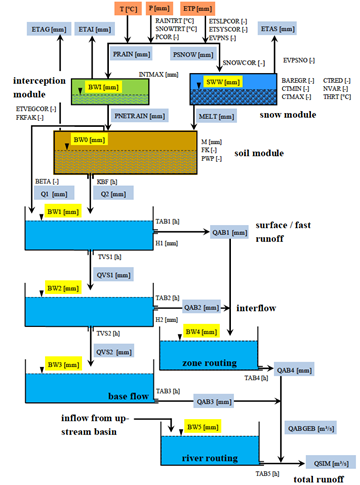
Figure 1: Schematic structure of the COSERO model (Herrnegger et al., 2005)
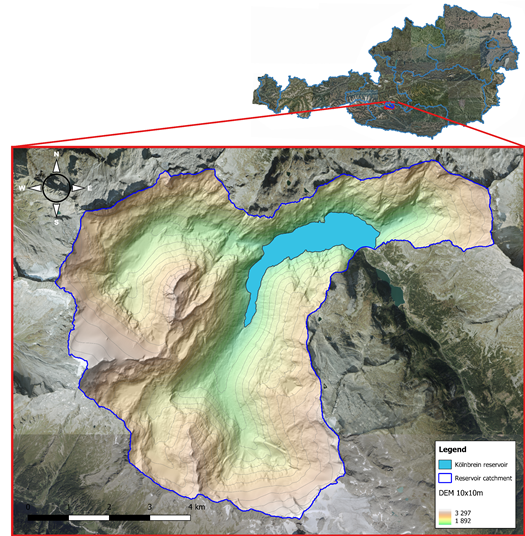
Figure 2: Topography of the headwater catchment of the Kölnbrein reservoir in the Malta Valley in Carinthia in Austria
References
Avanzi, F., Gabellani, S., Delogu, F., Silvestro, F., Cremonese, E., Morra di Cella, U., Ratto, S., Stevenin, H., 2021. S3M 5.1: a distributed cryospheric model with dry and wet snow, data assimilation, glacier mass balance, and debris-driven melt (preprint). Cryosphere. doi.org/10.5194/gmd-2021-92
Bartelt, P., Lehning, M., 2002. A physical SNOWPACK model for the Swiss avalanche warning. Cold Regions Science and Technology 35, 123–145. doi.org/10.1016/S0165-232X(02)00074-5
Feigl, M., Lebiedzinski, K., Herrnegger, M., Schulz, K., 2021. Machine-learning methods for stream water temperature prediction. Hydrol. Earth Syst. Sci. 25, 2951–2977. doi.org/10.5194/hess-25-2951-2021
Haiden, T., Kann, A., Wittmann, C., Pistotnik, G., Bica, B., Gruber, C., 2011. The Integrated Nowcasting through Comprehensive Analysis (INCA) System and Its Validation over the Eastern Alpine Region. Weather and Forecasting 26, 166–183. doi.org/10.1175/2010WAF2222451.1
Herrnegger, M., Senoner, T., Nachtnebel, H.-P., 2018. Adjustment of spatio-temporal precipitation patterns in a high Alpine environment. Journal of Hydrology 556, 913–921. doi.org/10.1016/j.jhydrol.2016.04.068
Kratzert, F., Klotz, D., Brenner, C., Schulz, K., Herrnegger, M., 2018. Rainfall-Runoff modelling using Long-Short-Term-Memory (LSTM) networks (preprint). Catchment hydrology/Modelling approaches. doi.org/10.5194/hess-2018-247
Kratzert, F., Klotz, D., Shalev, G., Klambauer, G., Hochreiter, S., Nearing, G., 2019. Towards learning universal, regional, and local hydrological behaviors via machine learning applied to large-sample datasets. Hydrol. Earth Syst. Sci. 23, 5089–5110. doi.org/10.5194/hess-23-5089-2019
Lehning, M., Völksch, I., Gustafsson, D., Nguyen, T.A., Stähli, M., Zappa, M., 2006. ALPINE3D: a detailed model of mountain surface processes and its application to snow hydrology. Hydrol. Process. 20, 2111–2128. doi.org/10.1002/hyp.6204
Nachtnebel, H.P., Baumung, S., Lettl, W., 1993. Abflußprognosemodell für das Einzugsgebiet der Enns und der Steyr. IWHW, BOKU Wien.
Wesemann, J., Herrnegger, M., Schulz, K., 2018. Hydrological modelling in the anthroposphere: predicting local runoff in a heavily modified high-alpine catchment. J. Mt. Sci. 15, 921–938. doi.org/10.1007/s11629-017-4587-5