Next level free-energy calculations
SUPERVISOR: Chris OOSTENBRINK
PROJECT ASSIGNED TO: Radek CRHA
The accurate prediction of the affinity between proteins and other biomolecules or small molecules is crucial for pharmaceutical and chemical companies during the drug design process or protein engineering. Nowadays, a bunch of computational methods is available to perform these predictions in sufficient quality for systems which can be correctly described by classical force fields. However, the parameterization of force fields for drugs and drug candidates is still not straightforward and a common source for inaccuracies. In this project, we will aim to develop methods that describe such molecules at a quantum mechanical level.
The project will focus on the development of a novel method of free-energy calculations. We will use deep neural networks to predict the energy of the given system on the quantum level. This approach should be much faster than common quantum calculations, with the added capability to describe the complicated chemistry of e.g. metalloproteins. Therefore, the affinity between proteins and potential drugs (or other molecules) should be calculated more accurately than was possible in the past. The performance of the method will be evaluated by comparison with experimental data provided by the industrial partners involved in the project.
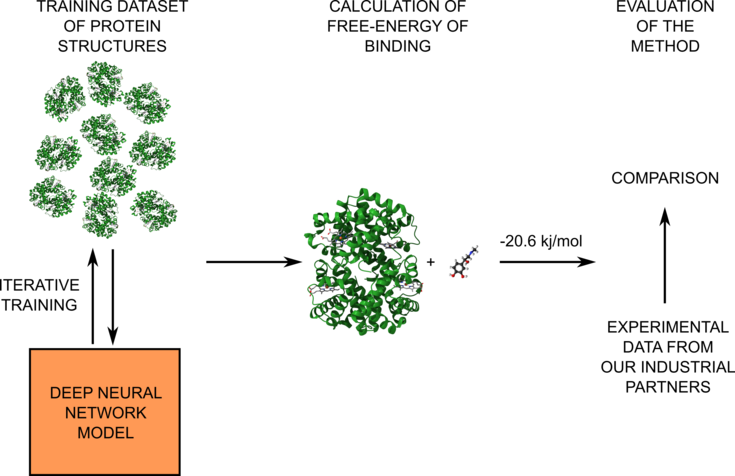
Figure 1: Schematic representation of the project workflow. In the first part of the project, we will train our deep neural network model on the QM data to predict accurate energies (left part). Then, the model will be used for the calculation of free-energy (middle part). Finally, the results will be compared with provided experimental data (right part).