Semi-automation of bioanalyses for real-time monitoring of quantity, purity and activity of biopharmaceuticals
SUPERVISOR: Alois JUNGBAUER
PROJECT ASSIGNED TO: Anna CHRISTLER
Process analytical technology enables real-time in-process control and product “quality by design” instead of “quality by testing” – a system that has been used in chemical industries for decades but only started recently to be implemented in the biopharmaceutical industry. We develop a monitoring system for real-time monitoring of protein quantity, purity and potency for downstream processes. On-line sensors are implemented to monitor the product stream quality. Statistical models are being derived from correlation of online signals with offline analytics. Additionally, we want to incorporate existing theoretical knowledge about dynamic protein adsorption to strengthen predictions and extend the operation field. Automated robust pooling of protein product is expected which enables parametric release of biopharmaceutical batches and also the operation of continuous production. In both cases, time and costs will be decreased significantly.
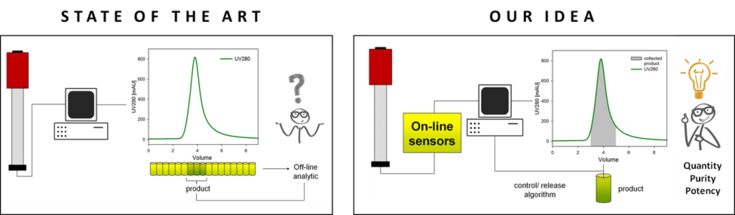
In the course of the PhD thesis, offline analytics will be semi-automated using a commercial liquid handling station. Results from manual and semi-automated procedures will be compared and evaluated by appropriate statistics to ensure continued consistency of data. We expect a substantial reduction in time and labour for biochemical analyses as well as an increase in reproducibility due to reduced operator influence. In the second part of the thesis, chromatographic processes will be described computationally by hybrid modelling techniques. Different algorithms have been shown by others to be successful for the optimization and control of complex upstream bioprocesses. One benefit of hybrid modelling is the possibility to balance the advantages and disadvantages of strictly data-driven and knowledge-based models. For example, unknown patterns can be detected by machine learning techniques while at the same time satisfying rational physical constraints and/or correlations.